Inspired by the working and cognitive mechanism of neurons, neuromorphic intelligence uses software and hardware co-design and computational modelling to achieve extreme power efficiency. Neuromorphic Technology can pave the way to smarter devices that augment human intelligence.
Neuromorphic Intelligence
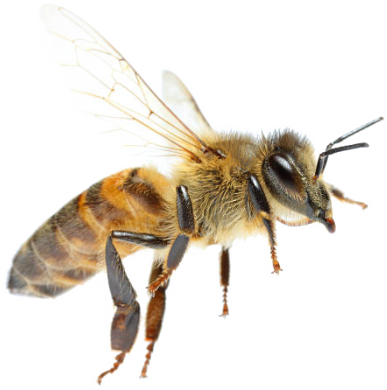
Ultimate AI Solutions
Insects can track, navigate, and avoid objects. They are capable of tasks such as inference, decision-making. In contrast, the human brain is far more complex and powerful.
As the basis of neuromorphic intelligence, neuromorphic chips imitate the functions of the brain, i.e., information processing, transmitting, and learning. With ultra-low power consumed, neuromorphic chips complete the tasks of sensing, learning, memorizing, decision-making, etc. making intelligence smarter.
Roadblocks lie ahead for AI
Hardware Bottlenecks
- Transistors are projected to reach their limits. The Moore’s law is coming to an end.
- In the face of tons of data, the Von Neumann architecture wastes cost and power on data transmission.
- The computational power is limited at the edge. The scenarios that require high computational power rely on cloud computing, The process of transmitting data between cloud and the edge wastes enormous power and cost.
Algorithm Bottlenecks
- Vast trove of data needs additional memory resources.
- A large-scale system requires computing resources and power.
- Applications of weak AI are restrained and are only available for limited tasks.
Features of Neuromorphic Chips
New Computing Mechanism
Event-driven computing that is based on sparse communication
New Architecture
Novel synchronize computing, distributed kernel/memory
Cutting Edge Algorithm
Spatial temporal computing using spiking neural network
Neuromorphic computing,
New Paradigm in Post-Moore era
Breakthrough in architecture and algorithm
Advantages
Event-driven
Power consumption reduced by 100-1000 times
Asynchronous
Real-time increased by 10-100 times
High temporal sequence dependency
Dynamic information processing
General Artificial Intelligence
Lower cost up to 100 times
Continuous Innovation with cloud-edge fusion solution
Sensor Node I
Low computational costs on the edge
AI computation nodes
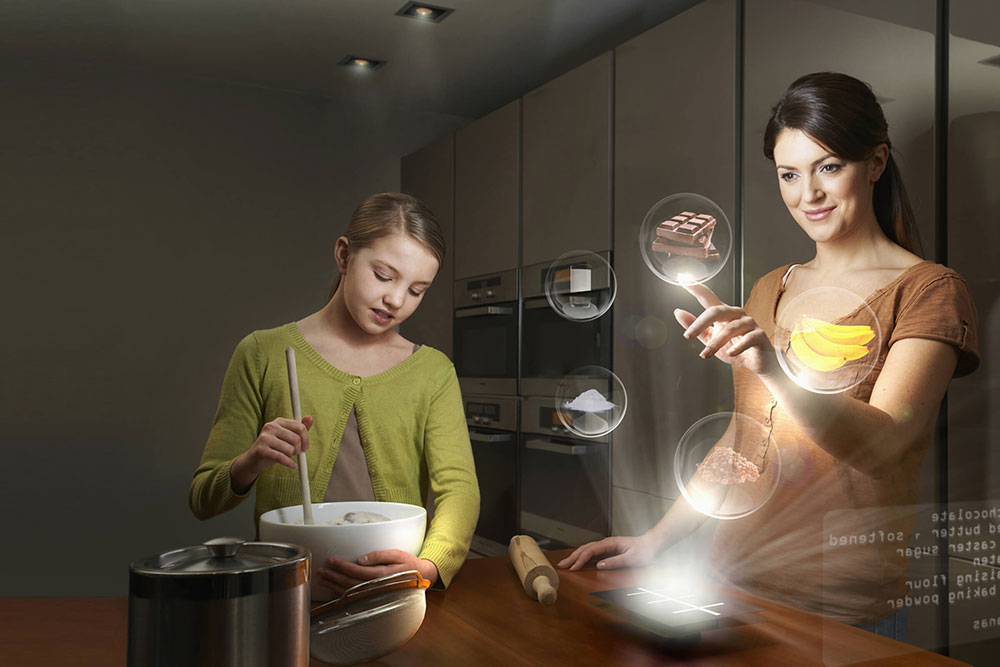
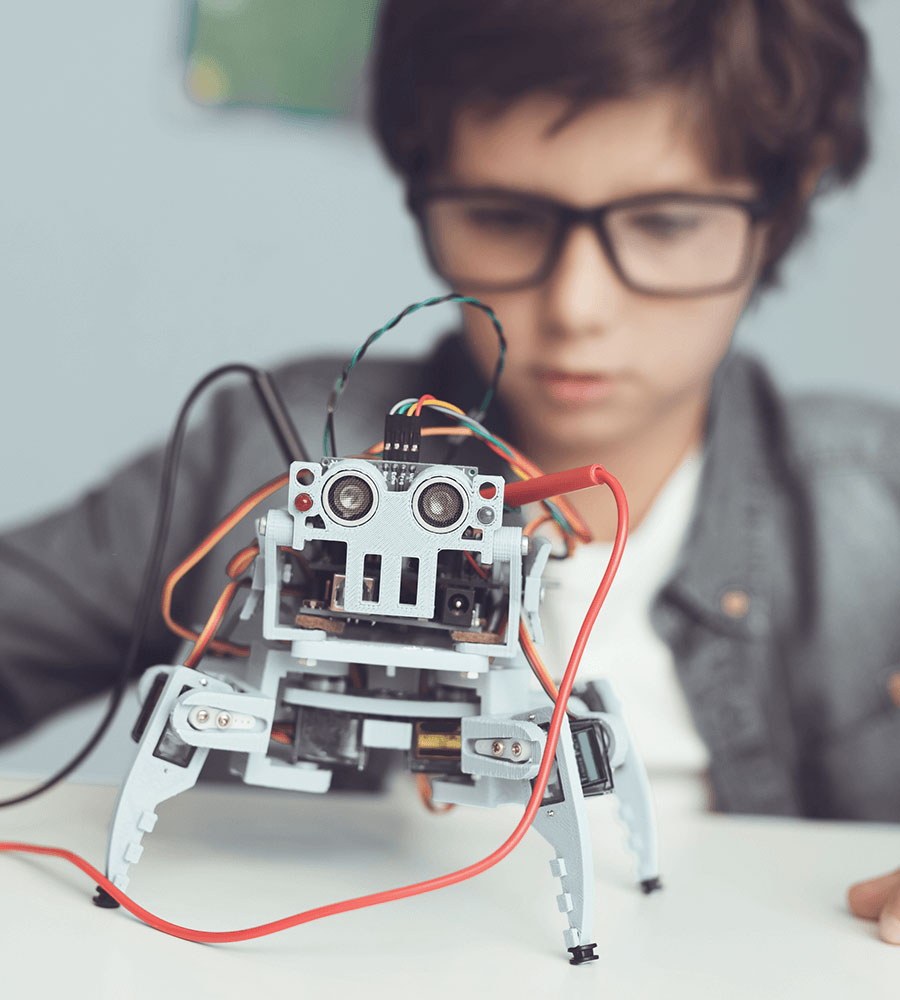
Sensor Node II
High computational costs on the edge
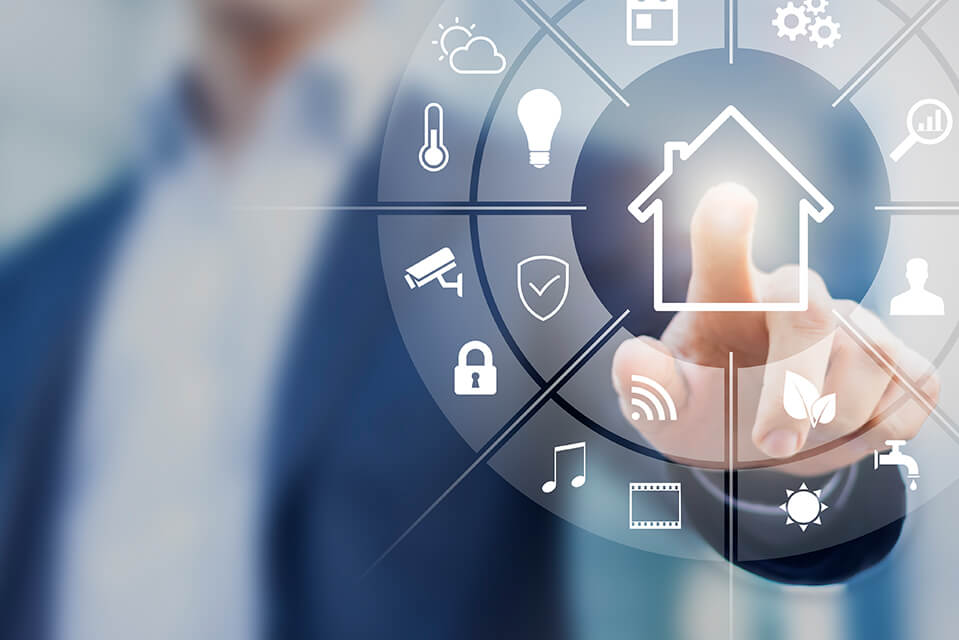
Sensor Fusion
Multi-sensory fusion computing
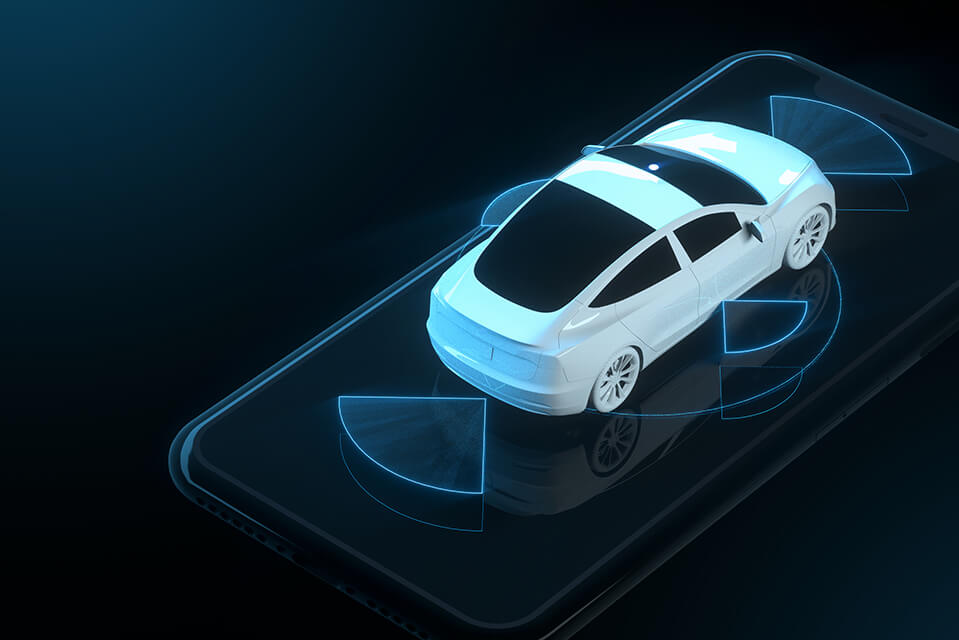
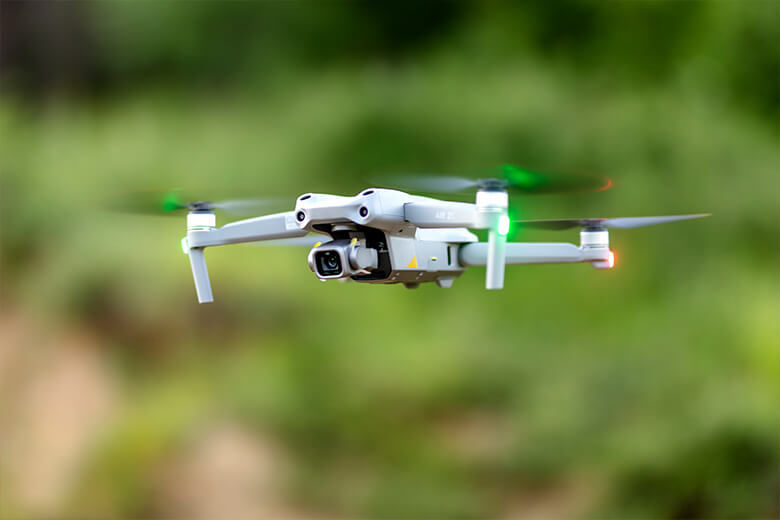
Edge Cloud
Analog computation, neuromorphic near-memory computing
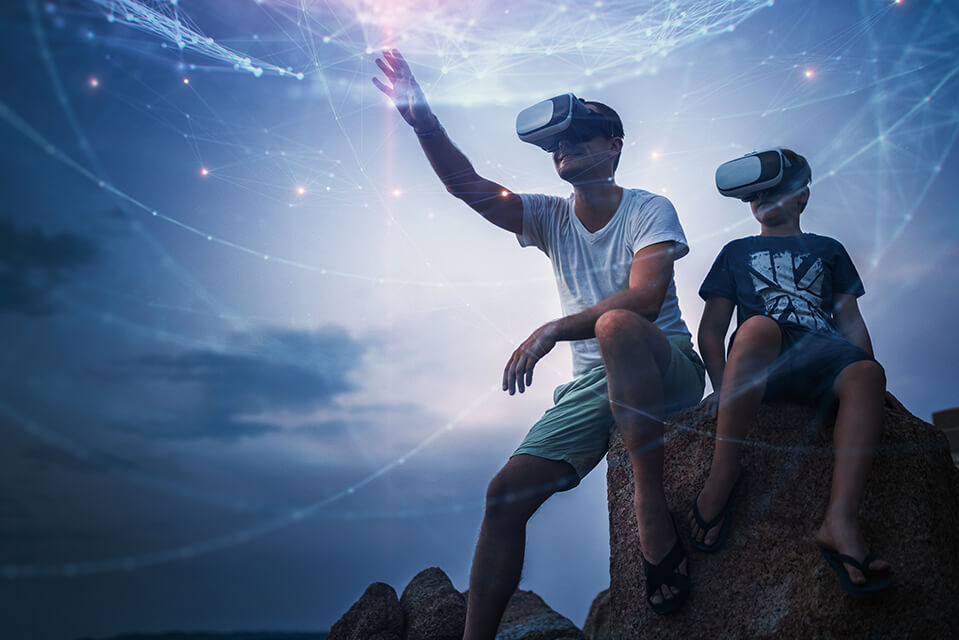
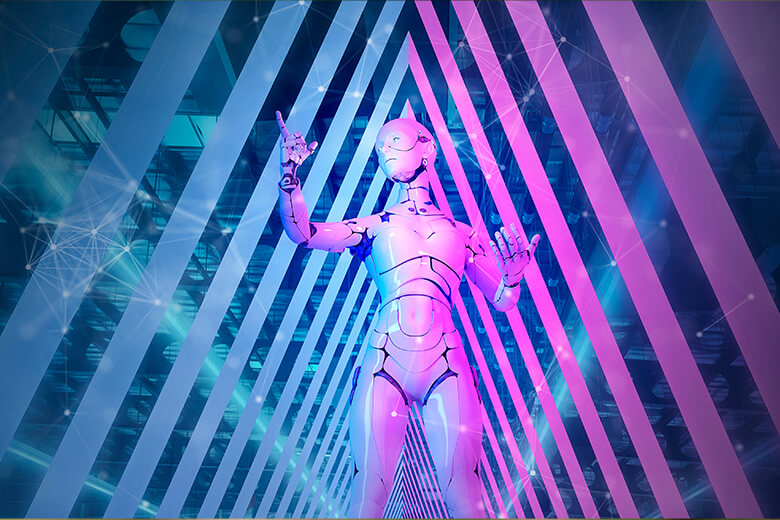