SynSense announces new support for training and deployment for ultra-low-power mixed-signal neuromorphic processors as part of their open-source Rockpool toolchain
SynSense announced new support for training and deployment for ultra-low-power mixed-signal neuromorphic processors as part of their open-source Rockpool toolchain (rockpool.ai).
Dynap™SE2 is a prototype ultra-low-power mixed-signal neuromorphic SNN inference processor produced as a collaboration between the University of Zürich, ETH Zürich, and SynSense.
It is the second in a series of efficient low-power devices designed to mimic features of biological neural processing. Each SE2 chip contains 1024 mixed-signal spiking neurons and 64k mixed-signal synapses.We have released for the first time a complete automated toolchain for training and deploying applications to Dynap™SE2.
Rockpool 2.6 provides a simple high-level API for training spiking neural networks and a detailed simulation of the dynamics of the low-power mixed-signal neurons on SE2.
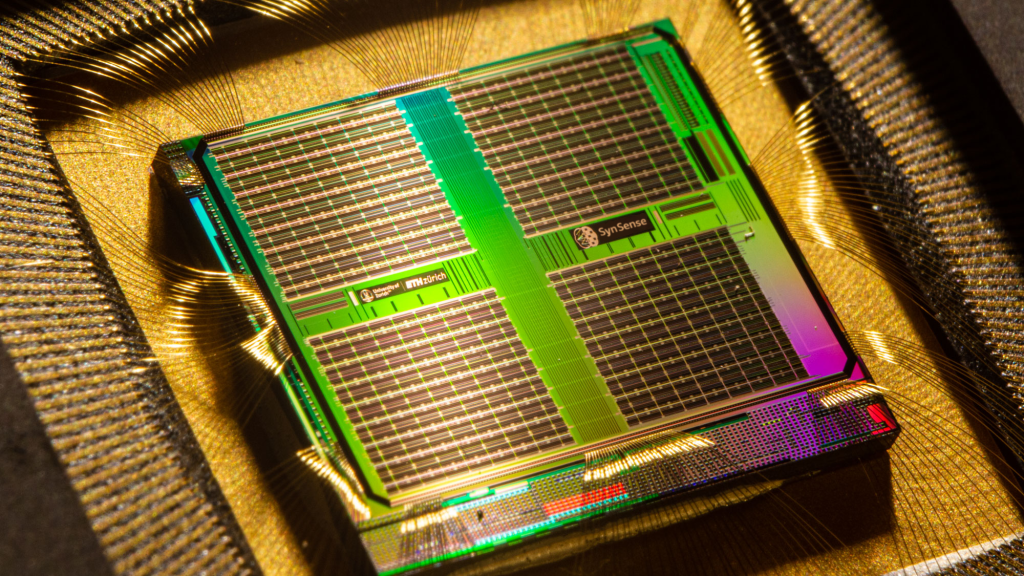
“It’s now possible to train SNN applications directly using the dynamics of the mixed-signal SE2 neurons,” said Uğurcan Çakal, lead developer on the SE2 tools. “It’s also easy to convert networks trained with other neuron models and deploy to SE2 devices.”
Rockpool handles quantisation of trained networks to the SE2 hardware constraints, as well as deployment and interfacing to SE2 development kit hardware.
“Our new tools bring the effort required to build ultra-low-power applications for mixed-signal neuromorphic devices from years down to months,” said Dylan Muir, Vice President for Global Research Operations at SynSense.
“We are making these tools available to the neuromorphic research community as part of our open-source software commitment. We hope this will help the community open up new use cases and new research applications for mixed-signal SNN processors. We can’t wait to see what they come up with!”